In today’s newsletter, you’ll use the OpenBB SDK to download news for a topic. Then, you’ll use OpenAI and build a prompt to predict the sentiment of a news headline. You’ll bring it all together with LangChain.
The result is a pandas DataFrame with a column of news headlines and a column with the predicted sentiment.
Let’s go!
Use OpenAI prompts for stock news sentiment
Generative Pre-trained Transformers (GPT) have significantly advanced the field of sentiment analysis by providing more nuanced and context-aware interpretations of text.
Their ability to capture long-range dependencies and understand context allows for a more accurate assessment of sentiment, reducing the likelihood of misclassification that is often seen in simpler models like Naive Bayes or Support Vector Machines.
The fine-tuning capabilities of these models enable domain-specific adaptations (like BloombergGPT or TimeGPT) which makes them useful for various applications in sentiment analysis.
In just a few lines of code, we can use the OpenAI GPT-4 model to predict the sentiment of a news headline.
Let’s get started!
Imports and set up
You’ll need an OpenAI API key to run this code. Once you have it, create a .env file in your working directly. Add the following line:
OPENAI_API_KEY=YOUR_API_KEY
Let’s import the OpenBB SDK for news and LangChain for building the app. Afterward, we instantiate an OpenAI GPT-4 LLM.
from openbb_terminal.sdk import openbb from langchain.chains import LLMChain from langchain.prompts import PromptTemplate from langchain.chat_models import ChatOpenAI from dotenv import load_dotenv load_dotenv() llm = ChatOpenAI(model="gpt-4", temperature=0)
Predict the sentiment
We use a straightforward prompt which outputs positive, negative, or neutral depending on the LLM’s interpretation of the sentiment.
prompt = """ Is the predominant sentiment in the following statement positive, negative, or neutral? --------- Statement: {statement} --------- Respond with one word in lowercase: positive, negative, or neutral. Sentiment: """ chain = LLMChain.from_string( llm=llm, template=prompt )
After creating the prompt, we create a LangChain chain using the OpenAI LLM and the prompt.
The LLMChain integrates a PromptTemplate, a Model, and Guardrails. It processes user input through formatting, submits it to the model for a response, and subsequently validates and corrects the model’s output as needed.
Next, we’ll download news headlines using the OpenBB SDK and apply the chain’s run method to each one.
msft = openbb.news(term="msft") msft["Sentiment"] = msft.Description.apply(chain.run) msft[["Description", "Sentiment"]]
This code creates a pandas DataFrame. We apply the chain.run method to the Description column and set the predicted sentiment in the Sentiment column.
Printing the output gives us a DataFrame with the predicted sentiment.
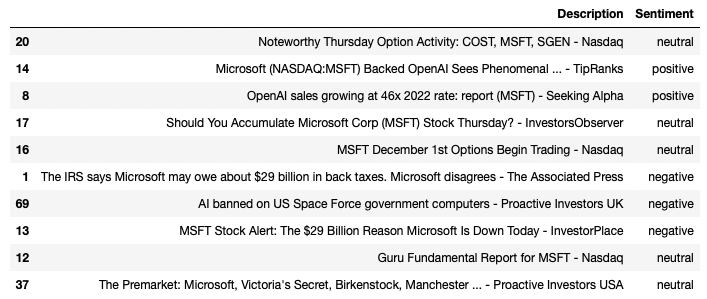
nextSteps
If you’re unfamiliar with prompt engineering, it’s the art of getting LLMs to return predictive, accurate results. As a next step, spend some time tweaking the prompt to improve it’s performance and predictability. For example, do you agree that MSFT’s $69B purchase of Activision is neutral?