Unveiling Option Pricing Methods
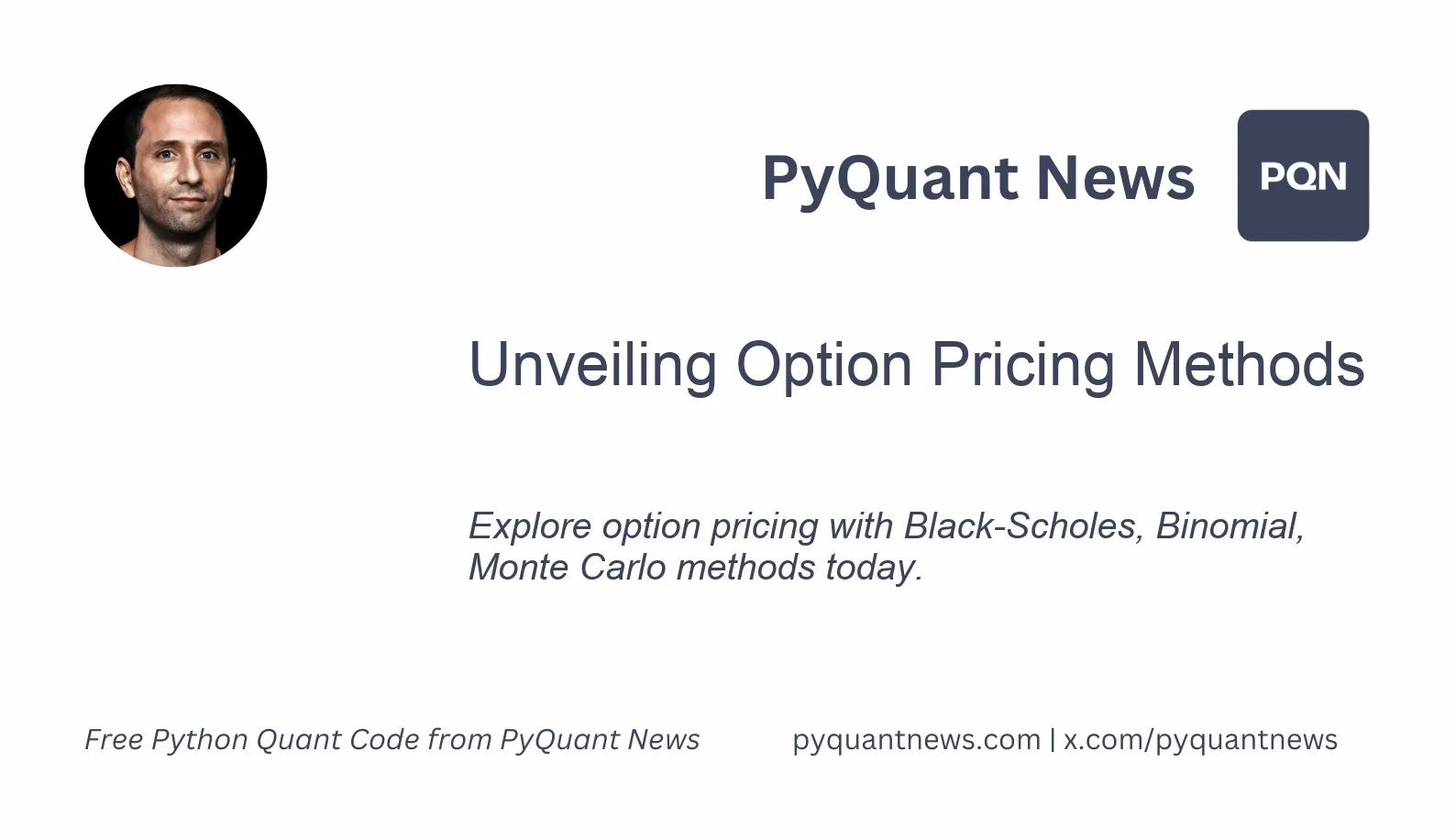
Unveiling Option Pricing Methods
In the intricate world of financial markets, options trading stands out as a sophisticated endeavor. Imagine a trader trying to price an option amidst volatile market conditions—understanding the right model can make or break their strategy. Among the myriad methods available, three have stood the test of time and scrutiny: the Black-Scholes model, the Binomial model, and the Monte Carlo simulation. Each of these approaches brings its own unique strengths and weaknesses, offering traders and financial analysts robust tools to understand the complexities of option pricing.
The Black-Scholes Model: A Milestone in Financial Engineering
Developed in 1973 by Fischer Black and Myron Scholes, with significant contribution from Robert Merton, the Black-Scholes model revolutionized the world of financial derivatives. The model provides a theoretical estimate of the price of European-style options and is grounded in the assumption of constant volatility and interest rates, which simplifies the mathematical complexity involved.
The Black-Scholes formula for a call option is:
[ C = S_0N(d_1) - Xe^{-rt}N(d_2) ]
Where:
- ( C ) is the call option price
- ( S_0 ) is the current stock price
- ( X ) is the strike price
- ( r ) is the risk-free interest rate
- ( t ) is the time to maturity
- ( N ) is the cumulative distribution function of the standard normal distribution
- ( d_1 = \frac{\ln(S_0 / X) + (r + \sigma^2 / 2) t}{\sigma \sqrt{t}} )
- ( d_2 = d_1 - \sigma \sqrt{t} )
- ( \sigma ) is the stock volatility
For instance, ( S_0 ) represents the current stock price, ( X ) is the strike price, ( r ) is the risk-free interest rate, and ( t ) is the time to maturity. The terms ( d_1 ) and ( d_2 ) are intermediary calculations that incorporate stock volatility (( \sigma )) and time.
The elegance of the Black-Scholes model lies in its closed-form solution, which allows for quick and efficient calculation of option prices. However, the assumptions of constant volatility and interest rates, along with the exclusion of dividends, limit its real-world applicability. The Black-Scholes model assumes constant volatility and interest rates, which rarely hold true in dynamic real-world markets. This simplification can lead to pricing inaccuracies, especially for options on stocks with fluctuating volatility or during periods of economic change.
The Binomial Model: Flexibility Meets Simplicity
The Binomial model, introduced by Cox, Ross, and Rubinstein in 1979, offers a more intuitive and flexible approach to options pricing. It models the underlying asset price as a discrete-time stochastic process, creating a binomial tree to represent the possible paths the asset price can take over time.
The Binomial model calculates the option price by starting at the end—its expiration date—and working backward. At each step, it considers whether the option should be exercised or held based on the price movements.
The key equations in the Binomial model are:
- ( u = e^{\sigma \sqrt{\Delta t}} ) (up factor)
- ( d = \frac{1}{u} ) (down factor)
- ( p = \frac{e^{r \Delta t} - d}{u - d} ) (risk-neutral probability)
Where:
- ( \Delta t ) is the time step
- ( u ) and ( d ) represent the factors by which the price can go up or down in each time step
- ( p ) is the probability of an upward movement in a risk-neutral world
In the Binomial model, ( u ) and ( d ) represent the potential up and down movements in the stock price per time step, and ( p ) is the risk-neutral probability of an upward movement. These factors help create a lattice of possible future stock prices. The Binomial model's flexibility in handling varying assumptions about volatility and interest rates, as well as its ability to price American options, makes it a valuable tool for traders.
Monte Carlo Simulation: Harnessing the Power of Randomness
Monte Carlo simulation, named after the renowned casino in Monaco, leverages the power of randomness to price options. This method involves generating a large number of random paths for the underlying asset price, based on assumed probability distributions, to estimate the expected payoff of the option.
Monte Carlo simulation involves:
- Generating numerous potential future paths for the underlying asset price based on assumed probability distributions.
- Calculating the payoff of the option for each path.
- Averaging these payoffs and discounting them back to their present value to determine the option's price.
Monte Carlo simulation is particularly useful for pricing complex derivatives with path-dependent features, such as Asian options or barrier options. Its strength lies in its flexibility and ability to handle a wide range of assumptions and scenarios. However, the method can be computationally intensive and may require sophisticated software and hardware to implement effectively. Although highly flexible, Monte Carlo simulation is computationally demanding. It requires significant processing power and sophisticated software to handle the numerous simulations needed for accurate pricing.
Comparative Analysis: Strengths and Weaknesses
Each of these models offers unique advantages and challenges:
- Black-Scholes: Efficient and widely used for European options, but limited by its assumptions of constant volatility and interest rates.
- Binomial: Flexible and intuitive, suitable for American options, but can be computationally intensive for large time steps.
- Monte Carlo: Highly versatile and capable of handling complex derivatives, but requires significant computational resources.
In summary:
- Black-Scholes: Efficient for European options but limited by constant assumptions.
- Binomial: Flexible and suitable for American options but computationally intensive for large time steps.
- Monte Carlo: Versatile for complex derivatives but requires substantial computational resources.
Practical Application: When to Use Each Model
For example, a trader dealing with a European call option on a stable, non-dividend-paying stock might find the Black-Scholes model most efficient. Conversely, an analyst pricing an American put option on a stock with fluctuating volatility would benefit from the Binomial model. For exotic options like Asian options, Monte Carlo simulation is the preferred method due to its ability to handle path dependency.
- Black-Scholes: Best suited for European options on non-dividend-paying stocks where the assumptions of the model hold reasonably well.
- Binomial: Ideal for American options or when dealing with varying volatility and interest rates.
- Monte Carlo: The go-to method for complex derivatives with path-dependent features or when dealing with multiple sources of uncertainty.
Resources for Further Learning
For those looking to delve deeper into the world of options pricing, the following resources provide comprehensive insights and practical guidance:
- "Options, Futures, and Other Derivatives" by John C. Hull: This seminal textbook offers an in-depth exploration of derivatives markets and pricing models, including extensive coverage of the Black-Scholes, Binomial, and Monte Carlo methods.
- "Financial Engineering: Derivatives and Risk Management" by Robert L. Navin: This book provides a practical approach to financial engineering, with detailed explanations of various option pricing models and their applications.
- Coursera's "Financial Engineering and Risk Management" Specialization: Offered by Columbia University, this online course series covers the principles of financial engineering, including options pricing and risk management techniques.
- Wilmott Magazine and wilmott.com: A leading resource for quantitative finance professionals, offering articles, forums, and tools related to derivatives pricing and financial modeling.
- "Monte Carlo Methods in Financial Engineering" by Paul Glasserman: A comprehensive guide to Monte Carlo simulation techniques and their application in financial engineering, providing both theoretical foundations and practical implementations.
Conclusion
The Black-Scholes, Binomial, and Monte Carlo methods each offer distinct advantages and are indispensable tools for financial professionals. By understanding the strengths and limitations of each model, traders and analysts can make more informed decisions and understand option pricing with greater confidence. As financial markets evolve, these models will continue to adapt, driving innovation and precision in derivatives trading.