The Power of Statistical Arbitrage in Finance
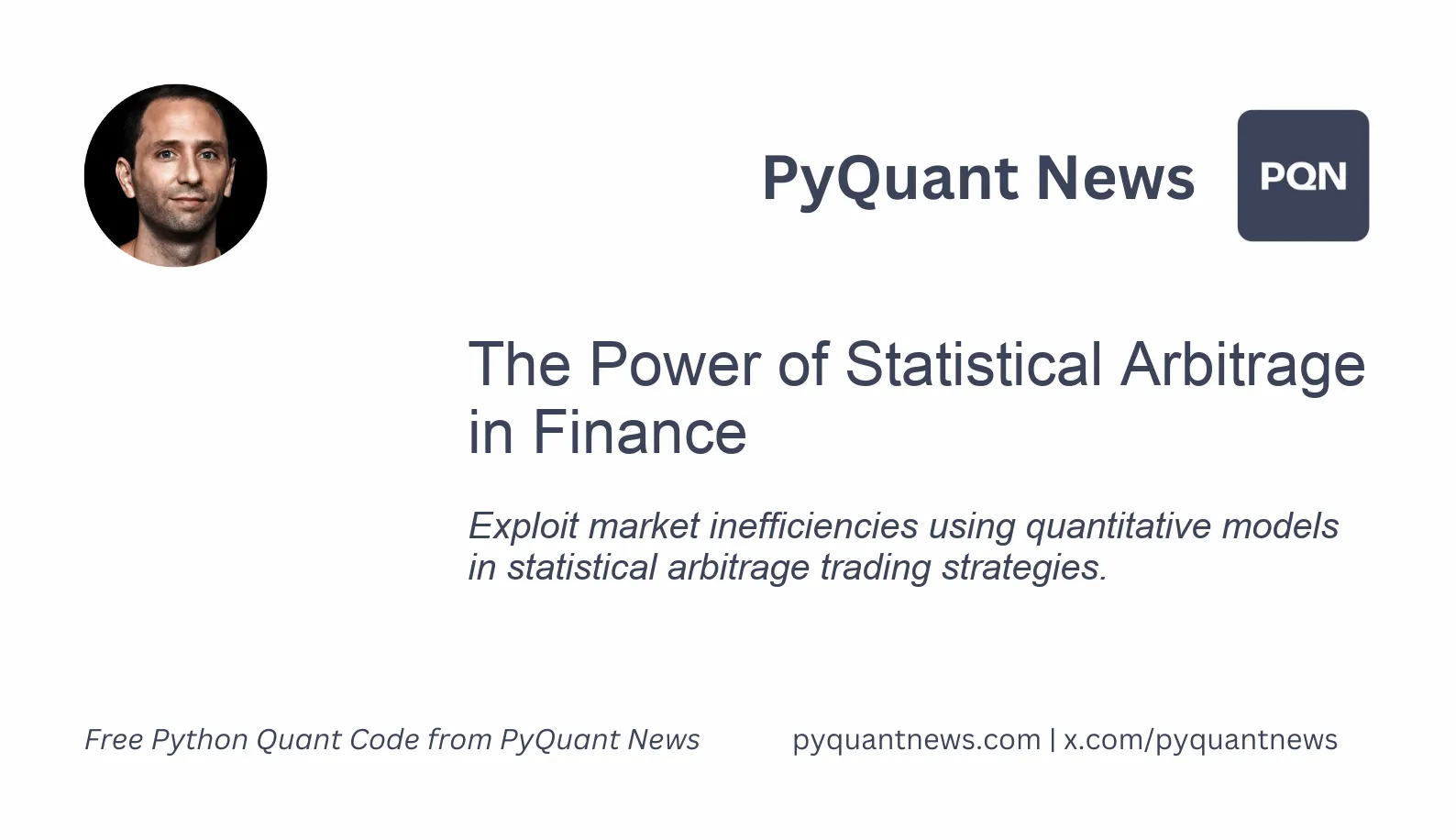
The Power of Statistical Arbitrage in Finance
In the unpredictable world of financial markets, the pursuit of consistent profitability is a common goal for traders and financial analysts. One powerful strategy that has emerged is statistical arbitrage. Known as "stat arb," this approach leverages statistical and mathematical models to identify and exploit market inefficiencies. This article delves into the mechanics, benefits, and limitations of statistical arbitrage, providing valuable insights for those looking to enhance their trading strategies.
Understanding Statistical Arbitrage
Statistical arbitrage, or "stat arb," relies on quantitative models to pinpoint temporary price discrepancies between related financial instruments. This strategy is rooted in the principle of mean reversion, suggesting that prices will eventually return to their historical averages. By capitalizing on these short-lived deviations, traders can potentially generate profits.
The Mechanics of Statistical Arbitrage
Statistical arbitrage operates through three primary steps:
- Data Collection and Analysis: Extensive historical price data for various financial instruments is gathered. This data is crucial for constructing statistical models that identify patterns and correlations.
- Model Development: Using techniques such as regression analysis, machine learning, and time series analysis, traders develop models predicting the expected price relationships between selected instruments. These models often include variables like volatility, volume, and economic indicators.
- Trade Execution: When a model identifies a price discrepancy, traders execute trades to exploit the inefficiency. This typically involves buying the undervalued instrument and selling the overvalued one, expecting prices to converge.
Key Strategies in Statistical Arbitrage
Several strategies fall under the statistical arbitrage umbrella, each with a unique approach:
- Pairs Trading: If the prices of two correlated stocks, like Coca-Cola and Pepsi, diverge from their historical relationship, a pairs trader might short the higher-performing stock (Coca-Cola) and go long on the underperforming stock (Pepsi), betting that their prices will converge.
- Market Neutral Strategies: These strategies aim to eliminate market risk by maintaining balanced long and short positions. This allows traders to profit from price discrepancies without exposure to broader market movements.
- Index Arbitrage: This strategy exploits price differences between an index and its constituent stocks. Traders buy or sell the index while taking offsetting positions in the underlying stocks to capture arbitrage opportunities.
- Convertible Arbitrage: Involves trading convertible securities (e.g., convertible bonds) against their underlying assets. Traders seek to profit from pricing inefficiencies between the convertible security and the stock it can be converted into.
Benefits of Statistical Arbitrage
The allure of statistical arbitrage lies in its potential to generate consistent returns while mitigating risk. Key advantages include:
- Risk Mitigation: By maintaining balanced long and short positions, traders can reduce exposure to market-wide movements, thereby minimizing risk.
- Diversification: Statistical arbitrage often involves trading multiple instruments across various asset classes, enhancing portfolio performance and reducing dependency on any single security.
- Data-Driven Decision Making: The reliance on quantitative models and extensive data analysis ensures that trading decisions are rooted in empirical evidence rather than subjective judgment.
- Scalability: Once a robust statistical arbitrage model is developed, it can be applied to different markets and instruments, allowing for scalability and potential expansion of trading activities.
Limitations and Challenges
Despite its advantages, statistical arbitrage presents challenges. Traders can mitigate these risks by employing several strategies:
- Model Risk: The accuracy of statistical models is paramount. Flawed models can lead to erroneous predictions and significant losses. Continuous refinement and validation are essential.
- Execution Risk: Timely execution is critical. Delays or slippage in trade execution can erode potential profits. High-frequency trading systems can help mitigate this risk.
- Market Regime Changes: Financial markets are dynamic and can undergo regime shifts that render historical relationships obsolete. Traders must adapt their models to changing market conditions by continuously updating their data and algorithms.
- Transaction Costs: Frequent trading can incur substantial transaction costs, including brokerage fees and bid-ask spreads, impacting overall profitability. Minimizing these costs through lower fees or more efficient trading algorithms is vital.
Resources for Further Learning
For those intrigued by statistical arbitrage, here are some valuable resources:
- "Statistical Arbitrage: Algorithmic Trading Insights and Techniques" by Andrew Pole: This comprehensive book covers both theoretical foundations and practical applications of statistical arbitrage strategies.
- "Quantitative Trading: How to Build Your Own Algorithmic Trading Business" by Ernest P. Chan: A practical guide to developing and implementing quantitative trading strategies, including statistical arbitrage.
- Coursera's "Financial Engineering and Risk Management" Course: Offered by Columbia University, this online course covers various aspects of quantitative finance, including statistical arbitrage, with hands-on experience in financial modeling.
- QuantConnect: An open-source algorithmic trading platform allowing traders to develop, test, and deploy statistical arbitrage strategies using historical data and various programming languages.
- "Algorithmic Trading and DMA: An Introduction to Direct Access Trading Strategies" by Barry Johnson: This book provides a comprehensive overview of algorithmic trading, including statistical arbitrage, and delves into the technical aspects of trading infrastructure.
Conclusion
Statistical arbitrage showcases the power of quantitative analysis in financial markets. By leveraging sophisticated models and data-driven insights, traders can identify and exploit market inefficiencies, potentially achieving consistent profitability. As financial markets continue to evolve, statistical arbitrage is poised to remain a vital trading strategy. Equip yourself with the knowledge and tools, and embark on the journey of statistical arbitrage.