The Future of Finance: AI in Dynamic Pricing
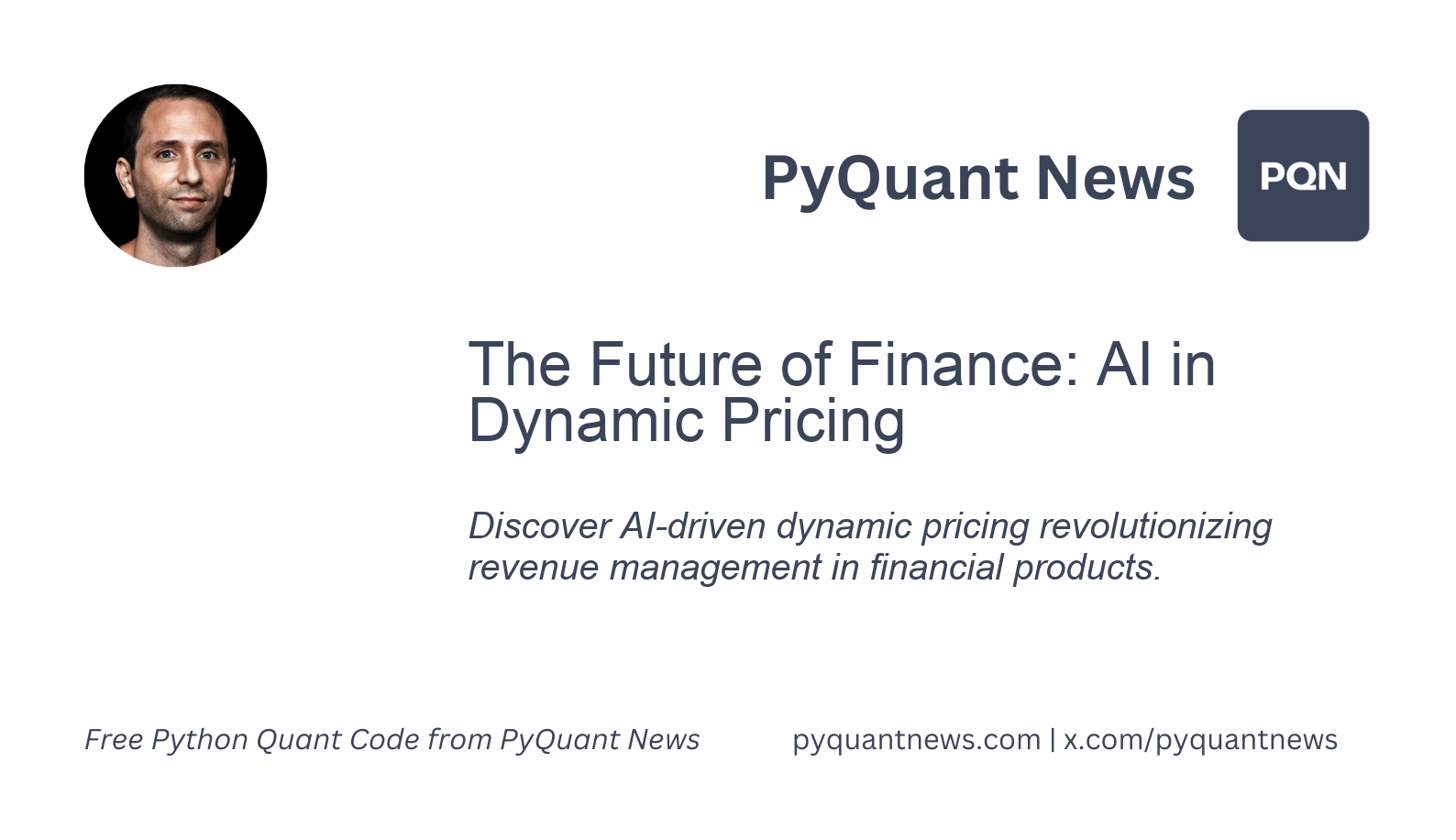
The Future of Finance: AI in Dynamic Pricing
In our increasingly digital world, the financial sector is on a constant quest to improve efficiency, optimize revenue, and offer personalized services to clients. One transformative development is the use of Artificial Intelligence (AI) in dynamic pricing and revenue management for financial products. This article explores how AI is revolutionizing these areas, the benefits and challenges it presents, and what the future holds for the financial industry.
The Rise of AI in Finance
Artificial Intelligence, with its ability to process vast amounts of data and identify intricate patterns, has found a natural home in the financial sector. From fraud detection to algorithmic trading, AI's applications are extensive. However, its role in dynamic pricing and revenue management is particularly groundbreaking, marking a significant departure from traditional pricing models that relied heavily on static data and manual adjustments. AI in finance is reshaping how financial institutions approach pricing and revenue optimization.
What is Dynamic Pricing?
Dynamic pricing refers to the strategy of adjusting prices in real-time based on market demand, competition, and other external factors. Traditionally, industries like retail, airlines, and hospitality have employed this approach. However, with the advent of AI, dynamic pricing has found its way into financial products such as loans, insurance policies, and investment services, offering a more responsive and competitive pricing model.
Revenue Management in Financial Products
Revenue management involves predicting consumer behavior at the micro-market level and optimizing product availability and pricing to maximize revenue growth. In the context of financial products, this could mean adjusting interest rates on loans, fees on credit cards, or premiums on insurance policies based on real-time market data and individual client profiles. AI-driven revenue management enables financial institutions to make these adjustments more accurately and efficiently.
The Mechanics of AI-Driven Dynamic Pricing
Data Collection and Analysis
AI systems thrive on data. For dynamic pricing and revenue management, the first step involves collecting vast amounts of data from various sources, including market trends, economic indicators, customer behavior, and even social media sentiments. Machine learning algorithms then analyze this data to uncover patterns and predict future market movements.
Real-Time Adjustments
Once the AI has processed the data, it can make real-time pricing adjustments. For example, in the case of loans, AI can adjust interest rates based on the borrower's credit score, current market rates, and the competitive landscape. This ensures that the financial institution remains competitive while also maximizing its revenue.
Personalization
One of the standout features of AI-driven dynamic pricing is its ability to offer personalized pricing. By analyzing a customer's financial history, spending patterns, and risk profile, AI can tailor financial products to meet individual needs, thereby enhancing customer satisfaction and loyalty.
Case Studies: AI in Action
Goldman Sachs' Marcus Platform
Goldman Sachs' Marcus platform is a prime example of AI-driven dynamic pricing in action. The platform uses sophisticated algorithms to offer personalized loan rates to customers. By analyzing a multitude of data points, Marcus can offer competitive rates while managing risk effectively, resulting in a significant increase in customer acquisition and retention rates.
PayPal's Dynamic Transaction Fees
PayPal has implemented AI to dynamically adjust transaction fees for its users. By analyzing transaction volumes, user profiles, and market conditions, PayPal can offer competitive fees to attract more users while maximizing its revenue. This approach has led to a notable increase in transaction volume and user engagement.
Insurance Industry Transformation
The insurance sector has also embraced AI for dynamic pricing. Companies like Lemonade use AI to assess risk and determine premiums in real-time. This not only speeds up the underwriting process but also ensures that customers receive fair and competitive rates. As a result, Lemonade has reported faster processing times and higher customer satisfaction scores.
Benefits of AI-Driven Dynamic Pricing and Revenue Management
- Enhanced Competitiveness: AI-driven dynamic pricing ensures that financial institutions can offer the best rates and products, thereby attracting more customers.
- Increased Revenue: By optimizing prices based on real-time data, financial institutions can maximize their revenue, particularly in sectors like lending and insurance.
- Improved Customer Satisfaction: Personalized pricing means that customers receive offers tailored to their needs and risk profiles, enhancing satisfaction and fostering loyalty.
- Operational Efficiency: AI can automate many processes involved in pricing and revenue management, reducing manual intervention and increasing operational efficiency.
Challenges and Ethical Considerations
Data Privacy and Security
The use of AI in dynamic pricing relies heavily on data. Ensuring the privacy and security of this data is paramount. Financial institutions must invest in robust cybersecurity measures to protect customer data from breaches.
Algorithmic Bias
AI systems are only as good as the data they are trained on. If the data contains biases, the AI can perpetuate these biases, leading to unfair pricing. Regular audits and the implementation of fairness algorithms can help mitigate these risks.
Regulatory Compliance
The financial sector is heavily regulated. Implementing AI-driven dynamic pricing must comply with existing regulations, which can vary by region. Financial institutions must work closely with regulators to ensure compliance while leveraging AI's benefits.
Customer Trust
While AI offers numerous benefits, gaining customer trust can be challenging. Transparent communication about how AI is used and the benefits it offers can help build trust and alleviate concerns. Providing clear explanations and maintaining transparency in AI processes can foster greater customer trust.
The Future of AI in Financial Products
The integration of AI in dynamic pricing and revenue management is still in its early stages. However, the potential is enormous. As AI technology continues to evolve, we can expect even more sophisticated algorithms capable of processing larger datasets and offering more accurate predictions.
Autonomous Financial Services
In the future, we may see the rise of fully autonomous financial services where AI handles everything from customer acquisition to product delivery. This could revolutionize the financial sector, making it more efficient and customer-centric.
Enhanced Predictive Capabilities
Advancements in machine learning and data analytics will further enhance AI's predictive capabilities. This will allow financial institutions to anticipate market trends and customer behavior with greater accuracy, leading to more effective pricing strategies.
Greater Personalization
As AI becomes more adept at analyzing individual customer data, the level of personalization in financial products will increase. This could lead to highly customized financial solutions that cater to the unique needs of each customer.
Resources for Further Learning
For those interested in exploring the intersection of AI and financial products further, here are some valuable resources:
- "Artificial Intelligence in Finance" by Yves Hilpisch: This comprehensive book delves into the applications of AI and machine learning in finance, offering both theoretical insights and practical examples.
- "Machine Learning for Asset Managers" by Marcos López de Prado: Focused on the asset management industry, this book explores how machine learning can be leveraged for investment strategies, risk management, and more.
- The AI in Financial Services Podcast: Hosted by Amazon Web Services, this podcast features industry experts discussing the latest trends and developments in AI for financial services.
- "FinTech Innovation: From Robo-Advisors to Goal-Based Investing and Gamification" by Paolo Sironi: This book provides a deep dive into the innovative technologies transforming the financial sector, including AI-driven solutions.
- McKinsey & Company Reports on AI in Finance: McKinsey frequently publishes insightful reports on the impact of AI in various industries, including finance. Their reports offer valuable data and case studies.
Conclusion
The use of AI for dynamic pricing and revenue management in financial products represents a significant leap forward for the financial industry. While there are challenges to overcome, the benefits far outweigh the risks. As AI technology continues to evolve, it will undoubtedly reshape the financial landscape, offering more efficient, personalized, and competitive financial products. For financial institutions, embracing AI is not just an option; it is a necessity in the quest for innovation and growth. Financial institutions should actively invest in AI technologies and collaborate with regulatory bodies to ensure a smooth and ethical transition into this new era.