The Backbone of Quantitative Finance
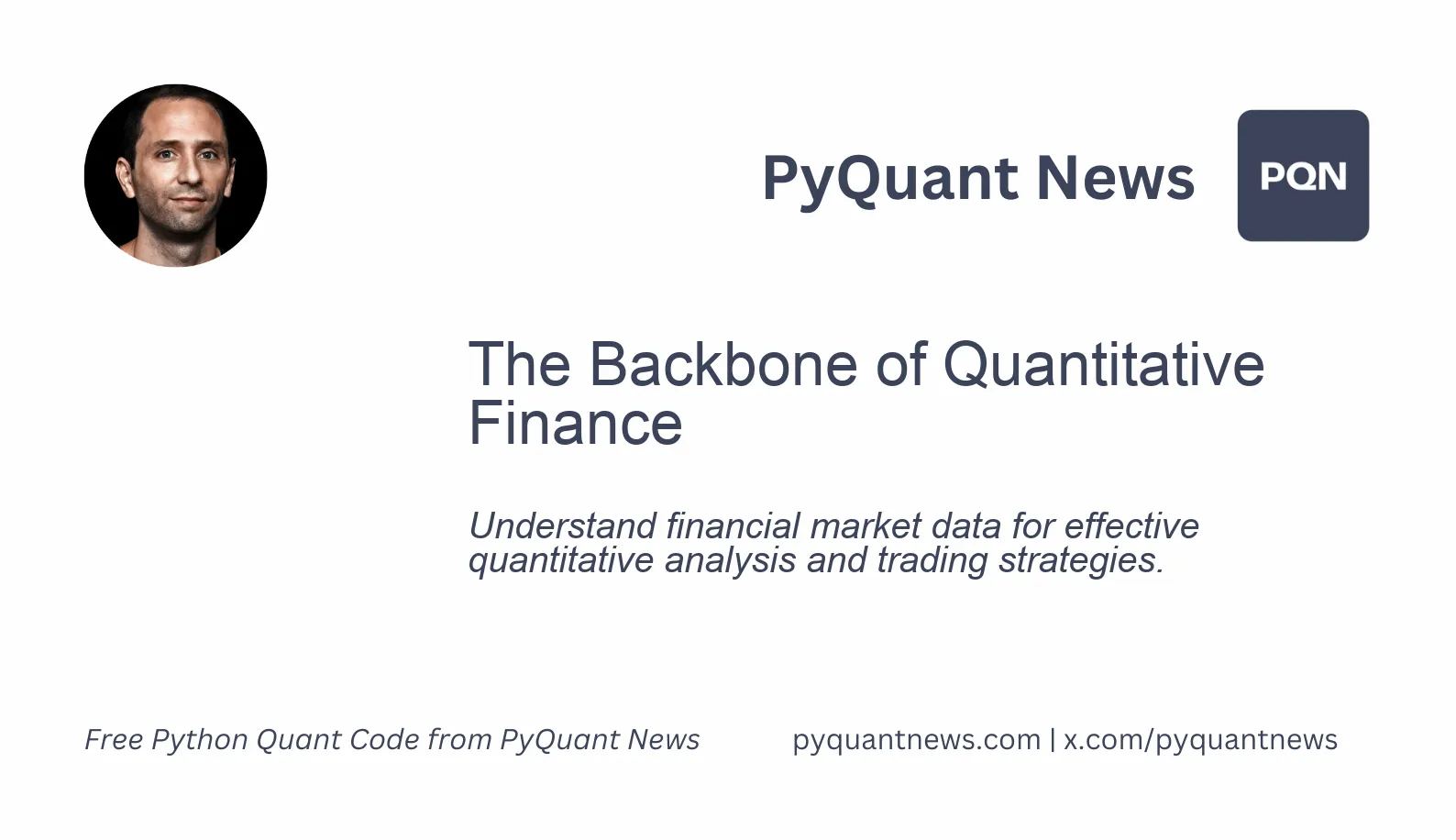
The Backbone of Quantitative Finance
In today's digital age, financial market data serves as the backbone of modern finance. The precision, speed, and accuracy of this data have transformed how investors, analysts, and institutions interact with the financial markets. For those involved in quantitative analysis, the value of financial market data cannot be overstated. This article explores the importance, sources, and role of financial market data in driving quantitative analysis.
What is Financial Market Data?
Financial market data includes a wide array of information related to the trading of financial instruments such as stocks, bonds, commodities, and derivatives. Key types of this data are:
- Price Data: Historical and real-time prices of financial instruments.
- Volume Data: The number of shares or contracts traded within a specific period.
- Fundamental Data: Financial statements, earnings reports, and balance sheets of companies.
- Economic Indicators: Macroeconomic data like GDP, unemployment rates, and inflation figures.
- Market Sentiment Data: News articles, social media posts, and analyst reports reflecting the mood and opinions of market participants.
Each type of financial market data shapes investment decisions and strategies in unique ways.
Financial Market Data in Quantitative Analysis
Quantitative analysis, or "quant" analysis, heavily relies on financial market data to develop mathematical models that predict market behavior and identify investment opportunities. Here's how this data is utilized:
1. Model Development
Quantitative analysts use historical price data to create models predicting future price movements. By analyzing patterns and trends, they aim to identify profitable trading strategies. For example, a quant might develop a mean-reversion model using historical price data, forecasting that prices will revert to their historical average over time.
2. Risk Management
Financial market data is crucial for assessing and managing risk. By analyzing historical price volatility and correlations between different assets, quants develop risk models to help investors minimize potential losses. Value-at-Risk (VaR) is a common risk metric derived from historical market data.
3. Algorithmic Trading
Algorithmic trading strategies rely on real-time market data to execute trades swiftly. These algorithms use data-driven models to identify arbitrage opportunities, execute trades, and manage positions without human intervention. High-frequency trading (HFT) firms, in particular, depend on the accuracy and speed of market data to gain a competitive edge.
4. Portfolio Optimization
Quantitative analysts leverage financial market data to construct and optimize investment portfolios. By analyzing the historical performance and correlations of different assets, they create diversified portfolios that aim to maximize returns while minimizing risk. Modern Portfolio Theory (MPT), developed by Harry Markowitz, is an example of how financial data is used for portfolio optimization.
5. Backtesting
Backtesting involves using historical market data to evaluate a trading strategy or model's performance. By simulating trades over past data, quants can determine their strategies' effectiveness before deploying them in live markets. This process helps refine models and eliminate unprofitable strategies.
Sources of Financial Market Data
Reliable financial market data is essential for accurate quantitative analysis. Primary sources include:
1. Stock Exchanges
Exchanges like the New York Stock Exchange (NYSE) and NASDAQ provide real-time and historical price and volume data for traded securities.
2. Data Providers
Companies such as Bloomberg, Thomson Reuters, and FactSet offer comprehensive financial data services, aggregating data from multiple sources, including exchanges, financial statements, and economic reports.
3. Government Agencies
Agencies like the Bureau of Economic Analysis (BEA) and the Federal Reserve release important economic indicators and reports, critical for macroeconomic analysis.
4. Financial News Outlets
Outlets like Bloomberg News, Reuters, and The Wall Street Journal provide real-time news and analysis that can impact market sentiment. Sentiment analysis tools often scrape data from these sources to gauge market mood.
5. Alternative Data Sources
Recently, alternative data sources such as social media, satellite imagery, and web traffic have gained prominence, offering unique insights for quantitative analysis.
Challenges in Using Financial Market Data
While financial market data is invaluable, it presents challenges like:
1. Data Quality
Ensuring data accuracy and reliability is paramount. Inaccurate or incomplete data can lead to erroneous conclusions and costly mistakes. Data providers employ rigorous validation processes to maintain data quality.
2. Data Integration
Integrating data from multiple sources can be complex and time-consuming. Different data sets may have varying formats, time zones, and conventions, requiring significant effort to harmonize.
3. Data Volume
The volume of financial market data can be overwhelming. High-frequency trading firms, for example, deal with terabytes of data daily. Efficient data storage, processing, and retrieval are crucial for handling such large volumes.
4. Latency
For real-time trading strategies, low-latency data is essential. Even microseconds of delay can impact trading performance. Quants invest heavily in technology to minimize data latency.
The Future of Financial Market Data
The landscape of financial market data is constantly evolving with trends like:
1. Big Data and Machine Learning
The integration of big data and machine learning techniques is revolutionizing quantitative analysis. These technologies enable analysts to process vast amounts of data and uncover hidden patterns.
2. Alternative Data Sources
The use of alternative data sources is expanding. Satellite imagery, credit card transactions, and social media sentiment are examples of unconventional data sets that quants are leveraging.
3. Blockchain and Distributed Ledger Technology
Blockchain technology is poised to enhance the transparency and security of financial market data. Distributed ledgers can provide tamper-proof records of transactions, reducing data manipulation risks.
4. Regulatory Changes
Regulatory changes, such as the European Union’s General Data Protection Regulation (GDPR), are impacting how financial data is collected, stored, and used. Quants must navigate these regulations to ensure compliance.
Resources for Learning More
For those eager to dive deeper into financial market data and quantitative analysis, here are some valuable resources:
1. Books
- “Quantitative Trading: How to Build Your Own Algorithmic Trading Business” by Ernest P. Chan: Provides practical insights into developing and implementing quantitative trading strategies.
- “Algorithmic Trading: Winning Strategies and Their Rationale” by Ernie Chan: A comprehensive guide to algorithmic trading, covering various strategies and their underlying principles.
- “Financial Market Data and Preprocessing for Machine Learning” by Boris Adryan: Delves into the preprocessing techniques required to prepare financial market data for machine learning applications.
2. Online Courses
- Coursera’s “Machine Learning for Trading”: Offered by the New York Institute of Finance, covering the application of machine learning techniques to trading strategies.
- edX’s “Algorithmic Trading and Finance Models with Python, R, and Stata”: Teaches algorithmic trading using popular programming languages.
- Khan Academy’s “Finance and Capital Markets”: A free resource offering a thorough introduction to financial markets and instruments.
3. Websites and Blogs
- Investopedia: Offers a wealth of articles, tutorials, and definitions related to finance and investing.
- QuantStart: A blog and resource hub for quantitative traders and analysts, featuring articles, tutorials, and research papers.
- Seeking Alpha: Provides market analysis, news, and investment ideas from a community of contributors.
Conclusion
Financial market data is the lifeblood of quantitative analysis, forming the foundation for constructing sophisticated models and strategies. As technology continues to advance, the availability and utilization of financial data will expand, offering new opportunities and challenges for analysts and investors. By understanding and leveraging financial market data effectively, one can navigate the complexities of modern finance with greater precision and confidence.