Neural Networks in Finance: Risk and Fraud Management
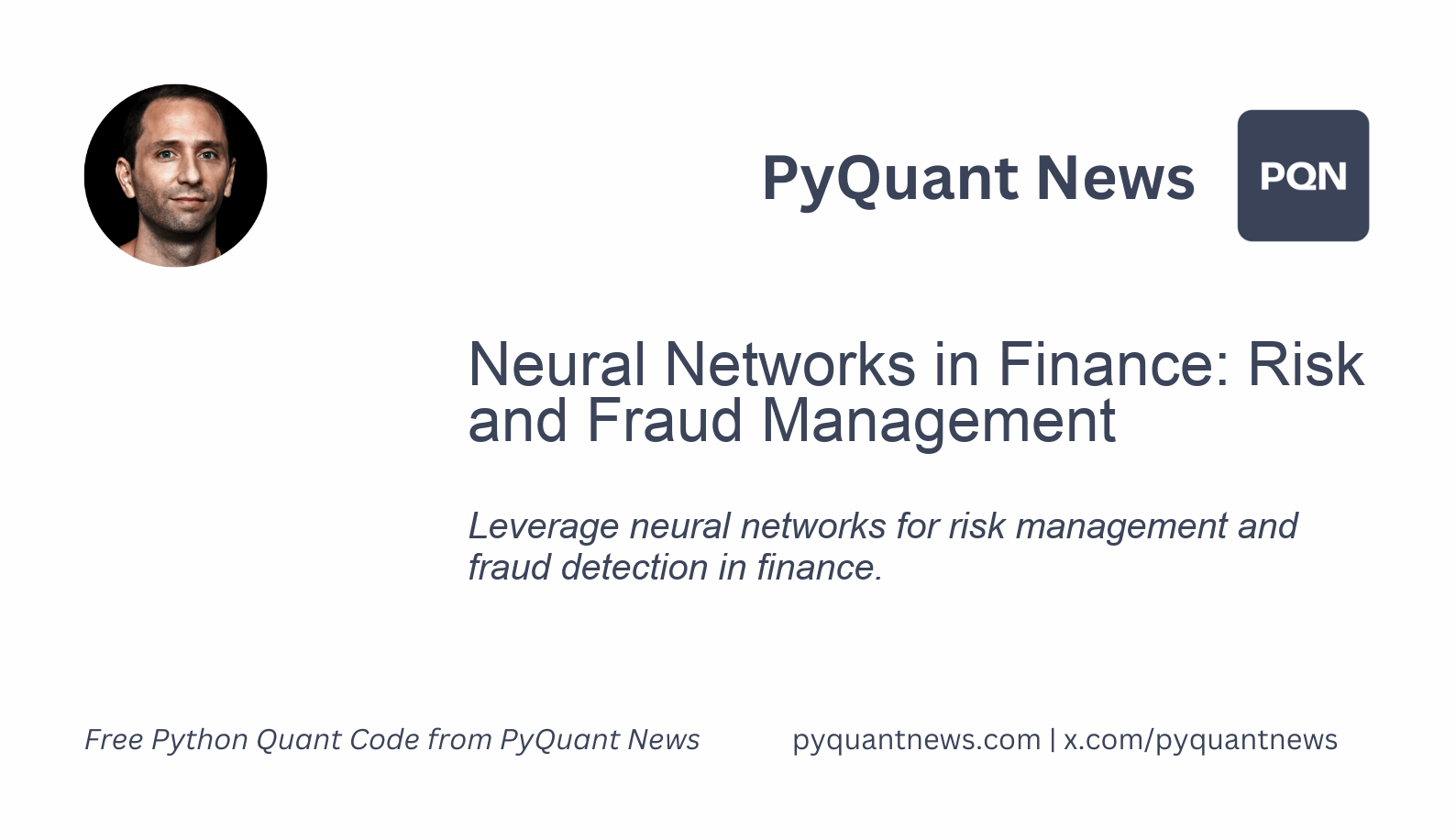
Neural Networks in Finance: Risk and Fraud Management
In the fast-paced world of financial institutions, maintaining trust and stability through effective risk management and fraud detection is more important than ever. As financial transactions increasingly shift to digital platforms, the complexity and volume of data have skyrocketed. Traditional methods are falling short, making way for neural networks—an advanced subset of artificial intelligence (AI)—to transform the financial industry. This article explores how neural networks enhance risk management and fraud detection, delving into their mechanisms, benefits, challenges, and future prospects.
The Rise of Neural Networks in Financial Institutions
Neural networks, inspired by the architecture of the human brain, consist of interconnected nodes (neurons) that excel in processing and analyzing data. These networks are exceptional at identifying patterns, making predictions, and learning from vast datasets. In financial institutions, neural networks are increasingly used to enhance risk management in finance and bolster fraud detection systems.
How Neural Networks Work
Neural networks consist of multiple layers: an input layer, one or more hidden layers, and an output layer. Each neuron in these layers receives inputs, processes them, and passes the output to the next layer, thereby facilitating complex computations.
For risk management in finance and fraud detection, neural networks are trained on vast datasets of historical transactions. By learning from past patterns of legitimate and fraudulent activities, these networks can identify anomalies and predict future risks with remarkable accuracy.
Risk Management: A New Paradigm
Neural networks present several advantages over traditional risk management methods, including:
- Data Processing Power: Neural networks can analyze massive datasets at high speed, uncovering patterns and correlations that might escape human analysts.
- Predictive Accuracy: By learning from historical data, neural networks can predict potential risks with a high degree of accuracy, allowing institutions to take proactive measures.
- Adaptability: Neural networks can adapt to new data and evolving market conditions, continuously improving their predictive capabilities.
Application in Credit Scoring
One of the most impactful applications of neural networks in financial institutions is credit scoring. Traditional models like the FICO score rely on a limited set of variables. In contrast, neural networks can analyze a broader range of data, including transaction history, social media activity, and even behavioral patterns. This holistic approach results in more accurate credit scores, reducing the risk of defaults and enabling financial institutions to extend credit to a broader customer base.
Stress Testing and Scenario Analysis
Stress testing and scenario analysis also benefit greatly from neural networks. By simulating various economic scenarios, these networks can predict the impact on an institution's portfolio, helping to identify vulnerabilities and develop contingency plans. This capability is particularly valuable in times of economic uncertainty, such as the COVID-19 pandemic, where traditional models may fall short.
Fraud Detection: Staying One Step Ahead
Another critical area benefiting substantially from neural networks is fraud detection. Financial fraud is a pervasive issue, costing institutions billions of dollars annually. Neural networks enhance fraud detection systems in several ways:
- Real-Time Detection: Neural networks can analyze transactions in real-time, identifying suspicious activities and flagging them for further investigation.
- Pattern Recognition: By learning from historical fraud patterns, neural networks can recognize subtle indicators of fraudulent behavior that might be missed by rule-based systems.
- Continuous Learning: Fraudsters continually evolve their tactics. Neural networks can adapt to new fraud patterns, ensuring that detection systems remain effective over time.
Transaction Monitoring
Neural networks are particularly effective in transaction monitoring for fraud. They can analyze a multitude of factors, such as transaction amount, location, frequency, and user behavior, to identify anomalies. For example, if a customer's spending pattern suddenly deviates from their usual behavior, the neural network can flag the transaction for further review. This proactive approach minimizes the window of opportunity for fraudsters and reduces financial losses.
Identity Verification
In addition to transaction monitoring, neural networks are used for identity verification. By analyzing biometric data, such as facial recognition and fingerprint scans, neural networks can authenticate users with high accuracy. This technology is especially valuable in preventing identity theft, a growing concern in the digital age.
Challenges and Considerations
Despite the numerous benefits, implementing neural networks in financial institutions comes with its own set of challenges:
- Data Quality: Neural networks require high-quality, labeled data for training. Incomplete or inaccurate data can lead to erroneous predictions.
- Interpretability: Neural networks are often described as "black boxes" because their decision-making processes are not easily interpretable. This lack of transparency can be a barrier to regulatory compliance and trust.
- Computational Resources: Training neural networks is computationally intensive and requires significant resources. Financial institutions must invest in robust infrastructure to support these systems.
- Bias and Fairness: Neural networks can inadvertently learn and perpetuate biases present in the training data. Ensuring fairness and avoiding discrimination in AI-driven decisions is a critical concern.
The Future of Neural Networks in Finance
The future of neural networks in financial institutions appears highly promising. As technology advances, we can expect to see even more sophisticated applications of neural networks in risk management and fraud detection. Some emerging trends include:
- Explainable AI: Efforts are underway to develop more interpretable neural network models, making it easier to understand and trust their decisions.
- Integration with Blockchain: Combining neural networks with blockchain technology can enhance transparency and security in financial transactions.
- Federated Learning: This technique allows neural networks to learn from decentralized data sources while preserving privacy, enabling more robust and secure models.
- Quantum Computing: The advent of quantum computing holds the potential to exponentially increase the computational power available for training neural networks, unlocking new possibilities for financial applications.
Resources for Further Learning
For those keen to delve deeper into the intersection of neural networks and finance, the following resources are invaluable:
- "Deep Learning" by Ian Goodfellow, Yoshua Bengio, and Aaron Courville: This comprehensive textbook covers the fundamentals of neural networks and deep learning, providing a solid foundation for understanding their applications in finance.
- Coursera's "AI for Everyone" by Andrew Ng: This online course, taught by one of the leading experts in AI, offers an accessible introduction to AI and neural networks, with insights into their practical applications.
- Kaggle: A platform for data science competitions, Kaggle hosts numerous projects related to financial data analysis, offering hands-on experience with neural networks and other machine learning techniques.
- "Machine Learning for Asset Managers" by Marcos López de Prado: This book focuses on the application of machine learning, including neural networks, in asset management, providing practical insights and case studies.
- Google AI Blog: This blog features articles and research papers on the latest advancements in AI and neural networks, including their applications in finance.
Conclusion
Neural networks are revolutionizing risk management and fraud detection in financial institutions by offering unprecedented accuracy, adaptability, and efficiency. While challenges remain, the potential benefits far outweigh the hurdles. As technology continues to evolve, neural networks will play an increasingly critical role in safeguarding the financial industry, ensuring its resilience in the face of emerging threats and uncertainties. Financial institutions that embrace this technology will be better equipped to maintain trust and stability in an ever-changing landscape.
By understanding and leveraging the power of neural networks, financial institutions can enhance their risk management and fraud detection capabilities and pave the way for a more secure and efficient financial ecosystem.