Exploring Factor Models in Financial Analysis
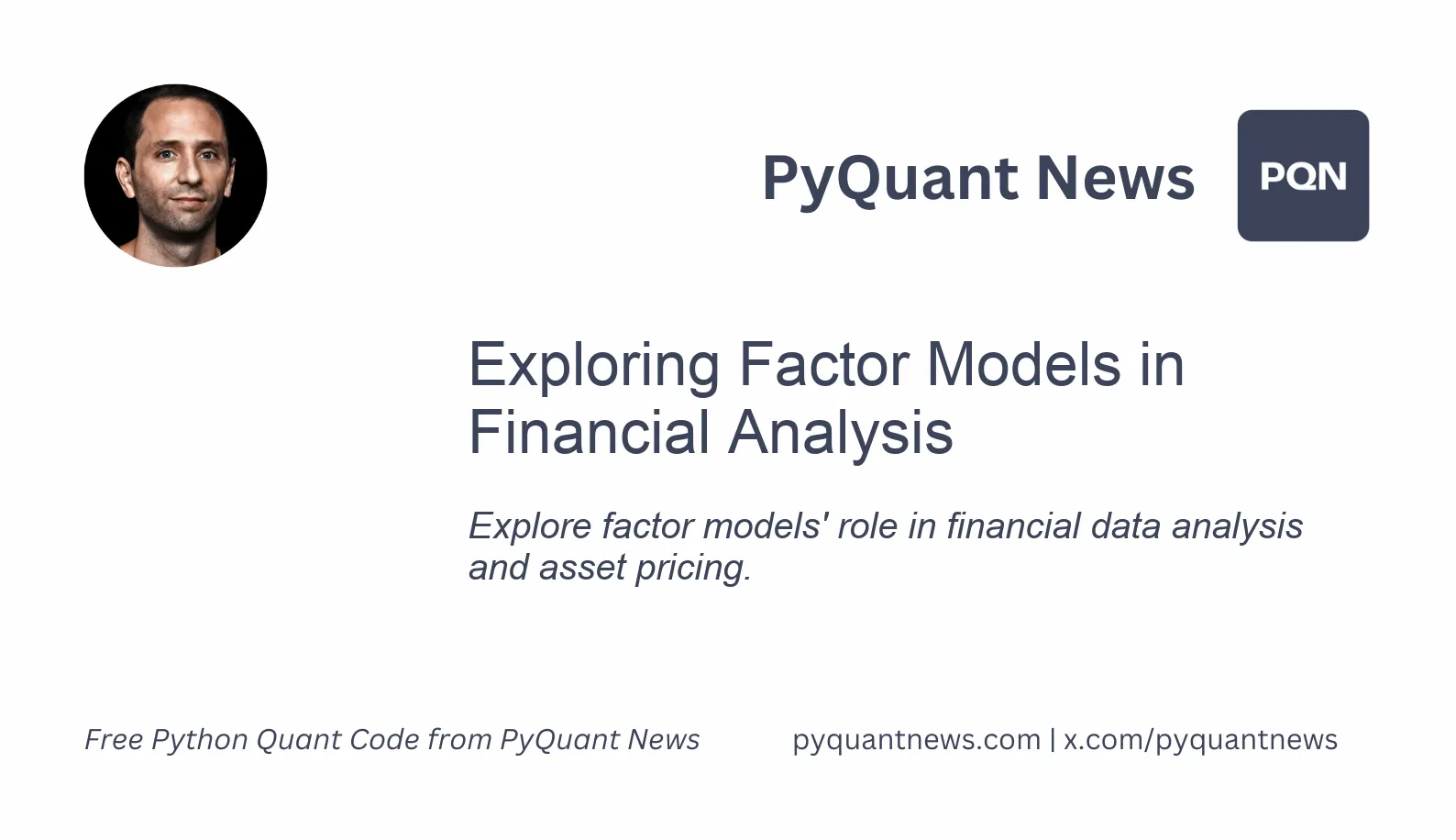
Exploring Factor Models in Financial Analysis
In the aftermath of the 2008 financial crisis, the need for robust financial analysis tools became apparent. Factor models emerged as essential instruments in understanding the complex dynamics of asset prices and market behavior. This article explores the essence of factor models, their types, applications, and the insights they bring to financial data analysis.
The Foundation of Factor Models
Factor models are quantitative tools used to explain the returns of a financial asset through exposure to various underlying risk factors. These models operate on the premise that asset returns can be broken down into a systematic component influenced by common factors and an idiosyncratic component unique to the specific asset.
Types of Factor Models
Single-Factor Models
Single-factor models explain the return of an asset by its sensitivity to a single factor, typically the market return. The Capital Asset Pricing Model (CAPM) is a prime example. CAPM posits that the expected return of an asset is a function of its beta (a measure of sensitivity to the market) and the risk-free rate.
Multi-Factor Models
Multi-factor models extend the single-factor approach by incorporating multiple factors that might influence asset returns. The Fama-French Three-Factor Model, which includes market risk, size risk, and value risk, is one of the most renowned multi-factor models.
Arbitrage Pricing Theory (APT)
Proposed by Stephen Ross, APT suggests that a variety of macroeconomic factors can influence asset returns. Unlike other models, APT does not specify which factors to include, allowing for a more flexible approach.
Statistical Factor Models
Statistical factor models, such as Principal Component Analysis (PCA), derive factors from the statistical properties of the data itself rather than relying on predefined economic factors.
Applications in Financial Data Analysis
Factor models have numerous applications in financial data analysis, spanning from portfolio management to risk assessment and beyond.
Portfolio Management
In portfolio management, factor models help in constructing diversified portfolios that optimize returns while managing risk. By understanding the different factors that drive returns, portfolio managers can:
- Identify Diversification Opportunities: Selecting assets with different factor exposures helps reduce unsystematic risk.
- Enhance Performance: Tilting portfolios towards factors expected to perform well can potentially enhance returns.
Risk Management
Factor models play a key role in risk management by identifying and mitigating various sources of risk:
- Understanding Systematic Risk: By breaking down the factors contributing to asset returns, managers can better understand the systematic risks affecting their portfolios.
- Stress Testing: Simulating how different factors might behave under various economic scenarios helps managers assess the potential impact on their portfolios.
Asset Pricing
Factor models provide a structured framework for understanding the pricing of assets:
- Explaining Anomalies: Traditional models like CAPM often fall short in explaining certain market anomalies. Multi-factor models offer more nuanced explanations for phenomena like the value premium or size effect.
- Informing Trading Strategies: Traders can use factor exposures to develop trading strategies that exploit mispricings or capitalize on expected factor movements.
Performance Attribution
Factor models enable detailed performance attribution, helping to identify the sources of returns:
- Breaking Down Returns: By attributing returns to different factors, investors can understand which factors contributed to performance.
- Benchmarking: Comparing portfolio performance against a benchmark composed of relevant factors provides insights into manager performance.
Real-World Examples
To illustrate the practical application of factor models, consider a few real-world scenarios:
Hedge Funds
Hedge funds often use factor models to identify and exploit market inefficiencies. For example, a fund might use a multi-factor model to identify undervalued stocks based on their exposure to various factors and construct a long-short strategy to profit from these insights.
Pension Funds
Pension funds use factor models to construct diversified portfolios that align with their risk tolerance and return objectives. By understanding factor exposures, they can better manage the risks associated with their liabilities.
Quantitative Asset Managers
Quantitative asset managers leverage statistical factor models to uncover hidden patterns in financial data. By applying techniques like PCA, they can identify latent factors that drive asset returns and develop systematic trading strategies.
Challenges and Considerations
While factor models offer significant benefits, they present challenges as well:
Factor Selection
Identifying the right factors is vital. Overfitting, where a model is too closely tailored to historical data, can lead to poor out-of-sample performance. This can be mitigated by using techniques such as cross-validation, which ensures that the model performs well on out-of-sample data.
Changing Dynamics
Financial markets are dynamic, and the relevance of factors can change over time. Continuous monitoring and updating of models are essential to ensure their effectiveness.
Data Quality
The accuracy of factor models heavily depends on the quality of the underlying data. Inaccurate or incomplete data can lead to misleading results.
Interpretation
Interpreting the results of factor models requires a deep understanding of both the statistical methods and the economic intuition behind the factors.
Resources for Further Learning
For those interested in delving deeper into factor models and their applications, several resources stand out:
- "Quantitative Equity Portfolio Management: Modern Techniques and Applications" by Ludwig B. Chincarini and Daehwan Kim: This book provides a comprehensive overview of quantitative investment strategies, including the use of factor models.
- "Active Portfolio Management: A Quantitative Approach for Producing Superior Returns and Controlling Risk" by Richard C. Grinold and Ronald N. Kahn: This classic text delves into the theory and practice of active portfolio management, with a strong emphasis on factor models.
- "Asset Pricing" by John H. Cochrane: Cochrane's book offers a rigorous exploration of asset pricing theory, including detailed discussions on factor models and their applications.
- Coursera's Financial Engineering and Risk Management Course: Offered by Columbia University, this online course covers various aspects of financial engineering, including the use of factor models in risk management.
- The Journal of Finance and The Review of Financial Studies: These leading academic journals regularly publish cutting-edge research on factor models and their applications, providing valuable insights for both academics and practitioners.
Conclusion
Factor models have significantly enhanced our ability to analyze financial data. They offer a structured approach to understanding market complexities. Their applications in portfolio management, risk assessment, and asset pricing underscore their indispensable role in modern finance. While challenges exist, the continued evolution of factor models promises to deepen our understanding of financial markets. For those eager to explore this fascinating field further, a wealth of resources awaits, offering the knowledge and tools needed to harness the power of factor models in financial data analysis.