Deep Learning Revolutionizes Financial Anomaly Detection
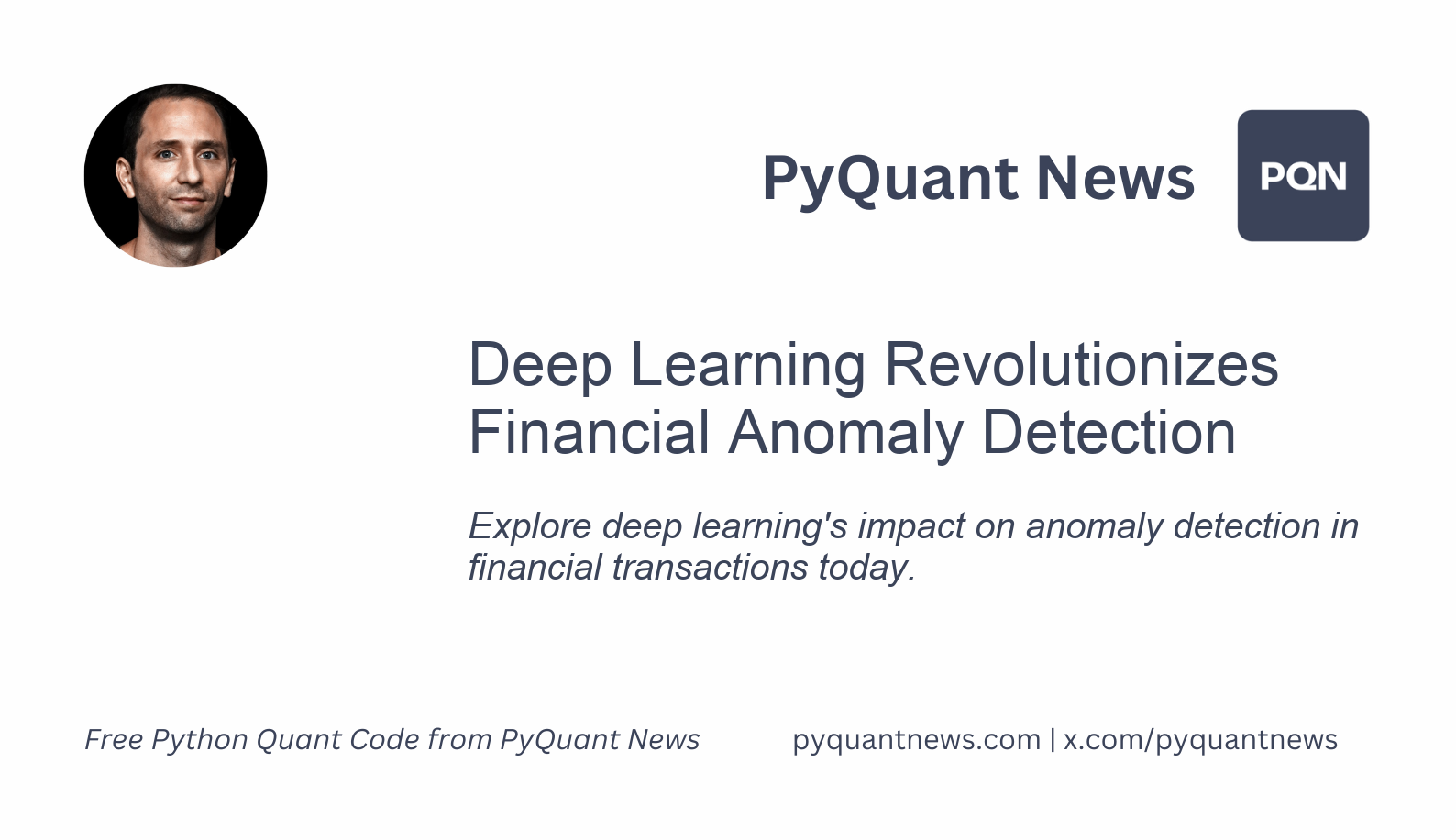
Deep Learning Revolutionizes Financial Anomaly Detection
In an era where financial fraud is increasingly sophisticated, combating it requires advanced tools. Deep learning, a subset of artificial intelligence (AI), is transforming anomaly detection in transaction data and financial statements. As financial institutions face the challenges of reducing false positives and enhancing detection accuracy, deep learning emerges as a promising solution. This article explores how deep learning techniques are revolutionizing anomaly detection, illustrating their potential and the methodologies behind them.
Understanding Anomaly Detection in Finance
Anomaly detection identifies rare events or observations that deviate significantly from the majority of the data. In finance, these anomalies often indicate fraudulent activities, errors, or other irregularities with significant repercussions.
Traditional methods of anomaly detection, including statistical analysis and rule-based systems, have historically served their purpose well. However, they are increasingly unable to keep pace with the complexity and volume of modern transaction data. Deep learning, with its ability to handle large datasets and discern intricate patterns, is changing the game.
How Deep Learning Works in Anomaly Detection
Deep learning models, particularly neural networks, mimic the human brain's ability to recognize patterns. These models learn from vast amounts of data, identifying subtle and complex relationships that traditional algorithms might miss.
Autoencoders
Autoencoders are unsupervised learning models effective for anomaly detection. They consist of two parts: an encoder that compresses the data into a lower-dimensional representation and a decoder that reconstructs the original data from this compressed form. The model is trained to minimize the reconstruction error—the difference between the input and the output. Once trained, it can highlight anomalies as those data points with high reconstruction errors, indicating they do not fit the learned pattern.
Recurrent Neural Networks (RNNs)
RNNs are well-suited for sequential data, such as transaction histories. They capture temporal dependencies and patterns in time-series data, making them particularly suitable for detecting anomalies in financial transactions. Long Short-Term Memory (LSTM) networks, a type of RNN, are adept at remembering long-term dependencies, essential for identifying subtle, long-term patterns indicative of fraud.
Generative Adversarial Networks (GANs)
GANs consist of two neural networks: a generator that creates synthetic data and a discriminator that evaluates the authenticity of the data. Through this adversarial process, the GAN improves its ability to distinguish between real and synthetic data. In the context of anomaly detection, the discriminator can identify anomalies as data points that diverge significantly from the generated data distribution.
Real-World Applications in Financial Transactions
Fraud Detection
Deep learning models can analyze transaction data to detect fraudulent activities in real time. By learning the normal behavior of individual accounts and comparing new transactions against this baseline, these models can flag unusual activities. For instance, if a credit card is suddenly used in a foreign country for a large purchase, the system can trigger an alert.
Financial Statement Analysis
Deep learning techniques can also scrutinize financial statements to identify inconsistencies and irregularities indicative of accounting fraud or financial distress. By analyzing historical financial data, these models can learn the typical patterns of revenue, expenses, and other financial metrics, flagging deviations that warrant further investigation.
Risk Management
In addition to fraud detection, anomaly detection plays a significant role in risk management. By identifying unusual market activities, deep learning models can help financial institutions mitigate risks associated with market manipulation, insider trading, and other illicit activities.
Challenges and Ethical Considerations
While deep learning offers powerful tools for anomaly detection, it is not without challenges. One major hurdle is the need for large, high-quality datasets to train the models effectively. Additionally, the black-box nature of deep learning models can make it difficult to interpret their decisions, raising concerns about transparency and accountability.
Ethical considerations are paramount, especially in the context of financial transactions. The deployment of deep learning models must ensure that individuals' privacy is protected and that the systems do not inadvertently reinforce biases present in the training data.
Future Prospects
The application of deep learning in anomaly detection is still in its early stages, with ongoing research and development promising to unlock even greater potential. Innovations such as explainable AI (XAI) aim to make deep learning models more transparent, while advances in federated learning promise to enhance privacy by enabling models to learn from decentralized data sources.
As the financial industry continues to embrace digital transformation, the integration of deep learning techniques for anomaly detection will undoubtedly become more pervasive. Financial institutions that leverage these technologies will be better equipped to safeguard against fraud, ensure compliance, and maintain the integrity of their financial systems.
Resources for Further Exploration
For those interested in delving deeper into the application of deep learning for anomaly detection in financial transactions, the following resources offer valuable insights:
"Deep Learning for Finance: A Guide to Financial Time Series Analysis and Prediction" by Jake Thompson.
This book provides a comprehensive introduction to deep learning techniques tailored specifically for financial applications, including anomaly detection in transaction data.
Coursera's "Deep Learning Specialization" by Andrew Ng.
This online course series offers a deep dive into various deep learning models, including practical implementations and case studies relevant to financial applications.
"Anomaly Detection: Principles and Algorithms" by Mehrotra et al.
This research paper explores various anomaly detection algorithms, including those based on deep learning, with a focus on their application in financial contexts.
Kaggle's "Credit Card Fraud Detection" Competition.
Kaggle's platform offers datasets and competitions that provide hands-on experience in developing and testing anomaly detection models for financial transactions.
The IEEE Computational Intelligence Society.
The IEEE Computational Intelligence Society publishes cutting-edge research on AI and deep learning, including applications in finance. Their journals and conferences are excellent resources for staying abreast of the latest developments in the field.
Conclusion
Deep learning is ushering in a new era of anomaly detection in the financial sector, offering unparalleled accuracy and efficiency in identifying fraudulent activities and financial irregularities. While challenges and ethical considerations remain, the potential benefits significantly outweigh the hurdles. As technology continues to advance, the integration of deep learning techniques will become indispensable for financial institutions striving to maintain security, compliance, and trust in an increasingly complex digital landscape.