AI Transforming Credit Scoring and Loan Approvals
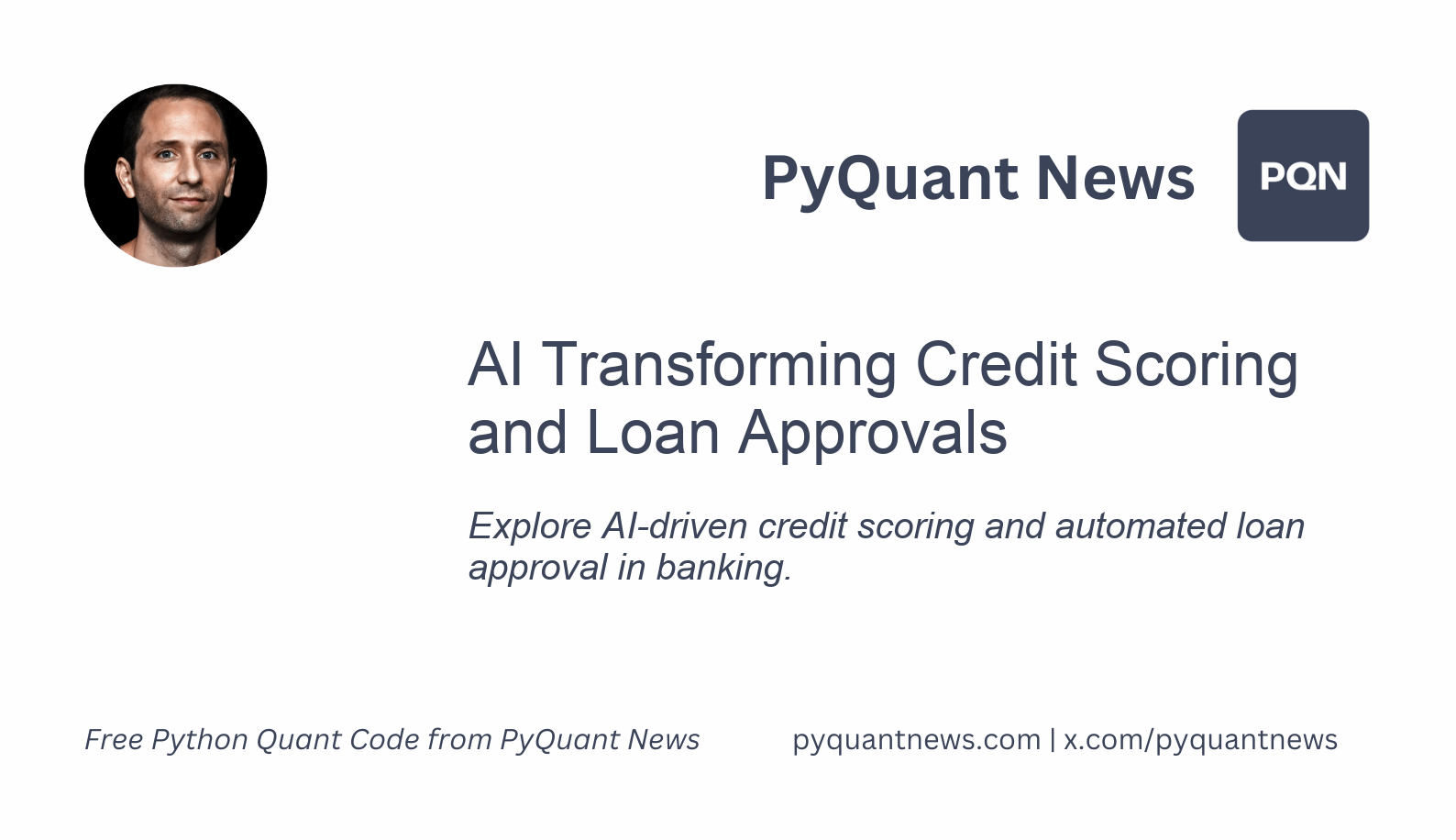
AI Transforming Credit Scoring and Loan Approvals
In the evolving landscape of financial services, AI in credit scoring and automated loan approval is becoming a transformative force. AI, leveraging machine learning algorithms to analyze data and make decisions, is increasingly adopted in the financial sector. Traditional methods, often cumbersome and biased, are being replaced by AI-driven credit scoring processes that promise greater efficiency, accuracy, and inclusivity. This article explores how AI is reshaping credit scoring and loan approval, highlighting both the benefits and challenges of this shift.
The Traditional Credit Scoring Paradigm
Credit scoring has historically relied on metrics such as credit history, outstanding debts, income levels, and employment status. The ubiquitous FICO score aggregates data from credit reports to produce a score that lenders use to assess a borrower’s creditworthiness. While effective to some extent, these traditional methods have notable limitations:
- Limited Data Utilization: Conventional models often overlook alternative data sources, such as utility payments, rental history, and even social media activity, which can provide a more holistic view of an individual’s financial behavior.
- Bias and Inequity: Traditional credit scores can inadvertently perpetuate socioeconomic and racial biases, heavily weighing factors that may not be equally accessible to all demographic groups.
- Static Nature: Credit scores are typically updated periodically rather than in real-time, potentially overlooking recent financial behaviors that could impact creditworthiness.
Enter AI: Revolutionizing Credit Scoring
AI-driven credit scoring is revolutionizing the industry by addressing these limitations through various means:
- Enhanced Data Integration: AI systems can analyze vast amounts of structured and unstructured data, including bank transactions, social media activity, and psychometric data, providing a comprehensive assessment of an individual's financial health. For example, AI can analyze bank transactions in real-time to detect spending patterns that indicate financial stability, which traditional methods might overlook.
- Dynamic and Real-Time Analysis: Machine learning models can continuously update scores based on real-time data, offering lenders a more current perspective on a borrower’s creditworthiness.
- Reduced Bias: By training on diverse datasets and employing fairness constraints, AI models can minimize biases inherent in traditional scoring systems, promoting greater financial inclusion.
Case Studies: AI in Action
Several financial institutions have successfully integrated AI into their credit scoring and loan approval processes, showcasing promising results.
Zest AI: This Los Angeles-based company uses machine learning to improve underwriting accuracy and expand credit access. By analyzing thousands of data points beyond the traditional credit report, Zest AI has demonstrated a reduction in loan defaults and an increase in loan approvals for underserved populations. Zest AI's approach highlights how enhanced data integration and reduced bias can lead to better lending decisions.
Upstart: Founded by ex-Google employees, Upstart uses AI to assess factors such as education, employment history, and standardized test scores. Their model has resulted in higher approval rates and lower interest rates for borrowers, while maintaining low default rates.
LenddoEFL: Operating in emerging markets, LenddoEFL leverages alternative data, including social media behavior and smartphone usage patterns, to assess credit risk. This approach has enabled them to provide credit to individuals who might otherwise be excluded by traditional scoring models.
The Loan Approval Process: AI’s Next Frontier
Beyond credit scoring, AI is also revolutionizing the loan approval process. Traditional loan approvals often involve manual verification and extensive back-and-forth communication, which can be time-consuming and prone to errors. AI streamlines this process through:
- Automated Document Verification: AI-powered systems can quickly verify the authenticity of documents such as pay stubs, tax returns, and bank statements, significantly reducing processing times.
- Risk Assessment: Machine learning models can assess borrower risk more accurately by analyzing a broader range of data points, including transaction patterns and behavioral data.
- Personalized Loan Offers: AI can tailor loan offers to individual borrowers based on their unique financial profiles, potentially offering better terms and interest rates.
Challenges and Ethical Considerations
While the benefits of AI in credit scoring and automated loan approval are compelling, several challenges and ethical considerations must be addressed:
- Data Privacy: The use of extensive personal data raises significant privacy concerns. Ensuring that data is collected and used in compliance with regulations such as GDPR and CCPA is essential.
- Algorithmic Bias: Despite efforts to minimize bias, AI models can still perpetuate existing disparities if not carefully designed and monitored. Continuous auditing and transparency are essential. For instance, a study by ProPublica found that an AI algorithm used in criminal sentencing was biased against African Americans, highlighting the importance of careful design and monitoring.
- Transparency and Explainability: AI-driven decisions can often be opaque, making it difficult for borrowers to understand why they were approved or denied. Enhancing the explainability of AI models is crucial for maintaining trust.
Future Outlook
The integration of AI into credit scoring and loan approval processes is still in its early stages, but the trajectory is clear. As algorithms become more sophisticated and data sources more diverse, the potential for AI to democratize access to credit and streamline lending processes will only grow. While the potential for AI in credit scoring and loan approval is immense, the industry must continue to prioritize ethical considerations and regulatory compliance to ensure a fair and equitable financial system.
Resources for Further Exploration
For readers interested in exploring this topic further, the following resources offer valuable insights:
- "Weapons of Math Destruction" by Cathy O'Neil: This book delves into the dangers of algorithmic bias and the impact of big data on society, providing a critical perspective on the use of AI in financial services.
- The Fairness, Accountability, and Transparency in Machine Learning (FAT/ML) Conference: An annual conference that explores ethical issues in machine learning, including bias and transparency, with a focus on ensuring equitable AI practices.
- The AI in Financial Services Special Interest Group (SIG) by the Financial Data and Technology Association (FDATA): This group offers reports and webinars on the latest developments in AI applications within the financial sector.
- "Prediction Machines: The Simple Economics of Artificial Intelligence" by Ajay Agrawal, Joshua Gans, and Avi Goldfarb: This book provides an accessible introduction to the economics of AI and its transformative potential across various industries, including finance.
- The AI Now Institute: A research institute dedicated to understanding the social implications of AI technologies, offering reports and policy recommendations on the ethical deployment of AI.
Conclusion
AI is poised to redefine the credit scoring and loan approval landscape, offering the promise of greater efficiency, inclusivity, and fairness. As financial institutions continue to embrace these technologies, they must collaborate with policymakers to create frameworks and guidelines that ensure ethical and effective deployment. With careful design, continuous monitoring, and a commitment to transparency, AI can indeed revolutionize the way we assess creditworthiness and approve loans, paving the way for a more equitable financial system.