AI Agents Revolutionizing Financial Trading Strategies
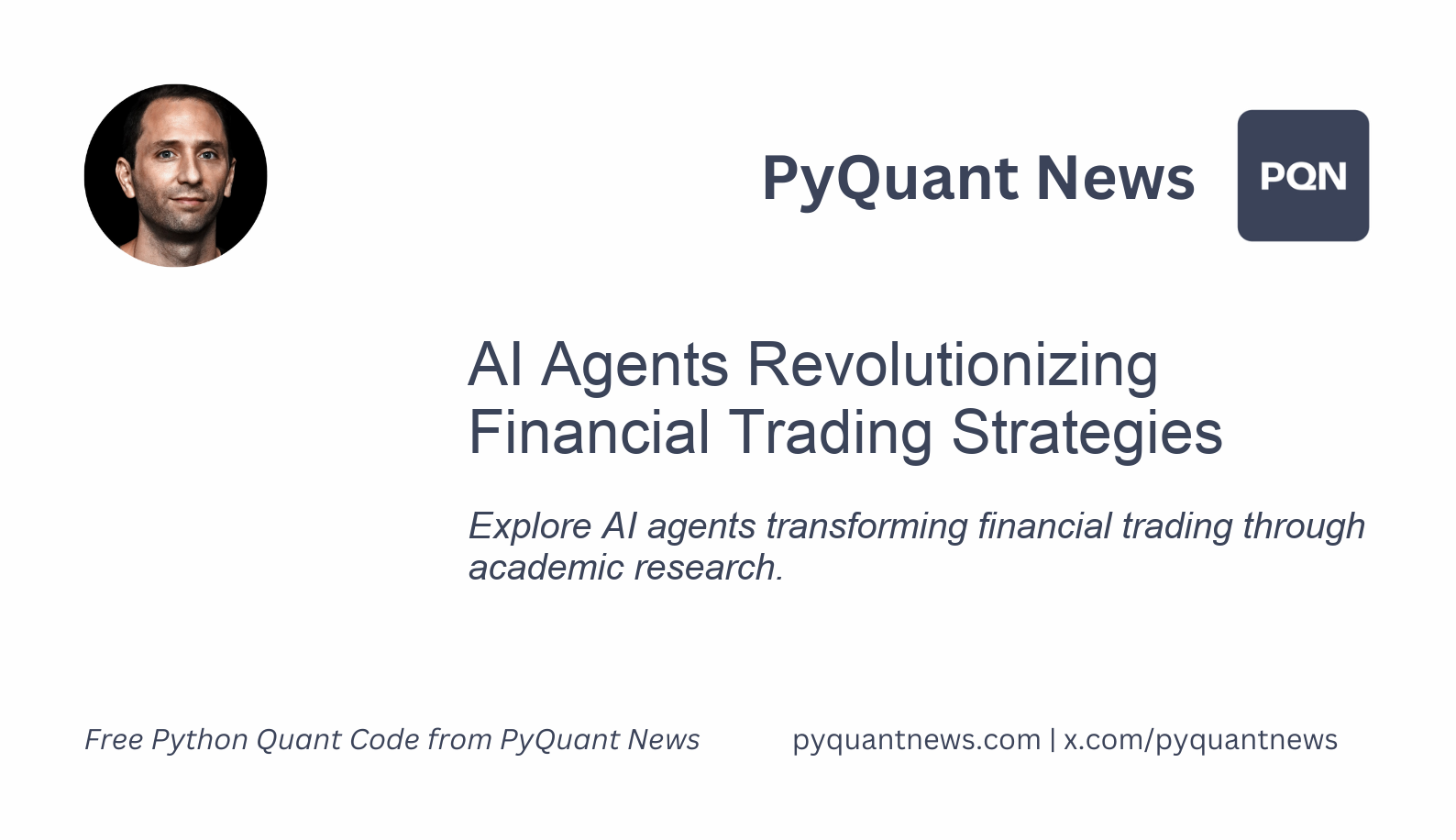
AI Agents Revolutionizing Financial Trading Strategies
The integration of AI agents in trading is transforming how financial trading strategies are developed, opening a new era where academic research in finance plays a pivotal role. This article dives into this evolution by exploring how AI agents utilize insights from academic research to revolutionize financial markets, promising both opportunities and challenges.
Transformation in Finance with AI
Combining artificial intelligence with financial markets is altering the landscape of financial trading strategies. Traditionally, technology has enhanced efficiency in financial markets, but AI agents bring something entirely new. These AI-driven systems analyze vast datasets, applying complex algorithms to extract actionable insights from academic research in finance. Traders can now seamlessly integrate sophisticated academic methodologies into their strategies.
Academic research often contains data-rich insights that can otherwise be challenging to decipher. AI agents in trading leverage natural language processing to bridge this gap, automating the extraction of information like statistical models and economic theories, and converting them into structured data to be utilized effectively.
Building Trading Strategies with AI
The process involves several key steps: data ingestion, strategy development, backtesting, and deployment—all essential for effective financial trading strategies.
Data Ingestion and Analysis
AI agents begin their work by processing academic research in finance from diverse sources. Natural language processing (NLP) is crucial here, enabling AI to understand and extract valuable financial insights. These advanced NLP models decode financial jargon and dense mathematical language, making complex academic content accessible.
Strategy Development
Using insights drawn from academic research in finance, AI agents utilize machine learning algorithms in trading to conceptualize innovative strategies. They identify key variables and run simulations to align predictive models with real-world market conditions.
Backtesting Trading Strategies
Before a strategy goes live, it must undergo rigorous backtesting. AI agents use historical data to assess the strategy's performance over time, ensuring robustness, identifying potential risks, and improving profitability.
Deployment in Live Markets
After backtesting, AI agents implement the strategies in live trading environments. They continuously adapt to market dynamics, leveraging continuous learning models to enhance strategies with new data, maintaining effectiveness in a volatile market.
Challenges with AI-Driven Trading Tools
Despite the optimism surrounding AI, several challenges in AI trading implementation must be acknowledged:
Data Quality and Practical Relevance
Academic papers sometimes involve outdated information, requiring AI agents to ensure accurate curation and validation.
Implementation Complexity
Converting academic insights into practical financial trading strategies demands both computational power and expertise.
Ethical and Regulatory Issues
AI models in financial markets raise concerns about transparency and bias, emphasizing the importance of regulatory compliance in AI finance.
Adapting to Market Volatility
Financial markets are inherently volatile, requiring AI-driven trading tools to adapt consistently.
The Future of AI in Financial Markets
The combination of AI agents in trading with academic research is ushering in a transformative era for financial markets. As AI technology advances, its capacity to interpret academic research in finance will redefine the industry, enhancing efficiency and making market access more inclusive.
Future collaborations between academia and the financial industry will drive the creation of advanced AI-driven trading tools. While human insight remains important, AI's analytical precision promises more informed, data-driven financial trading strategies.
Resources for Further Learning
To explore more about AI's role in finance:
- "Advancements in Deep Learning Applications for Finance" explores how deep learning tools can impact financial trading strategies.
- The Journal of Financial Econometrics provides insights into econometric techniques relevant to AI models in financial markets.
- Kaggle offers practical learning with datasets and notebooks for testing AI-driven trading tools.
- Coursera's "Machine Learning for Trading" course enhances practical skills for applying machine learning algorithms in trading.
- The CFA Institute Research Foundation merges academic insights with practical financial relevance.
Conclusion
AI agents, by integrating insights from academic research, are reshaping the development and implementation of financial trading strategies. This blend of AI and finance heralds an era of precision and adaptability. As technology evolves to meet current challenges, AI's role in transforming trading strategies will expand further. By leveraging AI agents, traders gain enhanced strategic insights and acumen, paving the way for future advancements in finance.